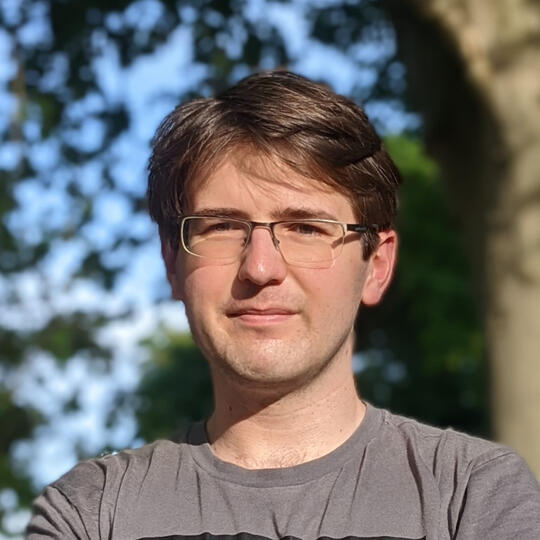
Jan Žemlička
Ph.D. candidate at UZH Finance and SFI
About
Hi! I am a Ph.D. candidate in Finance at the University of Zürich and the Swiss Finance Institute working under the guidance of Felix Kübler. Previously, I was a student of Marek Kapička at CERGE-EI, and spent Fall 2022 as a visiting scholar at UPenn Economics under the supervision of Jesús Fernandéz-Villaverde.My research interests lie in macrofinance, monetary economics, and development of computational methods for solving models originating in those fields.You can find my CV attached here.
RESEARCH
Intergenerational Consequences of Rare Disasters
with Marlon Azinovic
We analyze the intergenerational consequences of rare disasters in a calibrated overlapping generations model featuring realistic household portfolios and equilibrium asset prices. Households own houses and trade in bonds and equity. In a disaster, young households suffer from reduced labor income and tightened borrowing constraints. Older households lose a large portion of their savings invested in risky assets. The relative winners are households shortly before retirement, who have a more stable labor income, are not borrowing constrained, and young enough to benefit from large returns of assets purchased during the disaster at depressed prices. In order to solve the model, we advance contemporary deep learning based solution methods along two complementary dimensions. First, we introduce an economics-inspired neural network architecture that, by construction, ensures that market clearing conditions are always satisfied. Second, we illustrate how to solve models with multiple assets by introducing them step-wise into the economy. These two innovations enable us to reduce the number of equilibrium conditions, which are not fulfilled exactly, and to substantially improve the stability of the training algorithm.Presented at: Reading Group, UPenn (2022); CERGE-EI, Prague (2023); Advances in Computational Economics and Finance, UZH (2023); CEF Conference, Nice (2023); DSE Conference,+ Lausanne (2023); EEA-ESEM Conference, Barcelona (2023); Math Seminar, ETH Zurich; Brown Bag Seminar,+ John Hopkins University (2023); Macro Lunch Seminar,+ UPenn (2023). Seminar,+ Toronto Metropolitan University (2024); Seminar,+ University of Copenhagen (2024); Seminar,+ University of North Carolina at Chapel Hill, (2024); Seminar,+ ESSEC Paris (2024); Seminar,+ IE Madrid (2024); Seminar,+ University of Sussex (virtual), (2024); Seminar,+ National University of Singapore, (2024).+: Presented by coauthor
The methodological part of this paper was previously circulated as Economics-Inspired Neural Networks with Stabilizing Homotopies
Average Inflation Targeting in a Behavioral Heterogeneous Agent New Keynesian Model
with František Mašek
We analyze the optimal window length in the average inflation targeting rule within a Behavioral THANK model of Pfäuti and Seyrich (2022). The central bank faces an occasionally binding effective lower bound (ELB) or persistent supply shocks and can also use quantitative easing when we merge Pfäuti and Seyrich (2022) with Sims et al. (2020). We show that the optimal averaging period is infinitely long in the case of a conventional degree of myopia. However, finite yet long-lasting window lengths dominate for a higher cognitive discounting. We solve the model locally and globally to disentangle the effects of uncertainty about hitting the ELB in the future, leading to a downward inflation bias in the case of the global solution. Given the solution technique, the welfare loss difference is considerably decreasing in the degree of history dependence.
GET IN TOUCH
Department of Finance at the University of Zürich
Plattenstrasse 14
8032 Zürich
Switzerland[email protected]
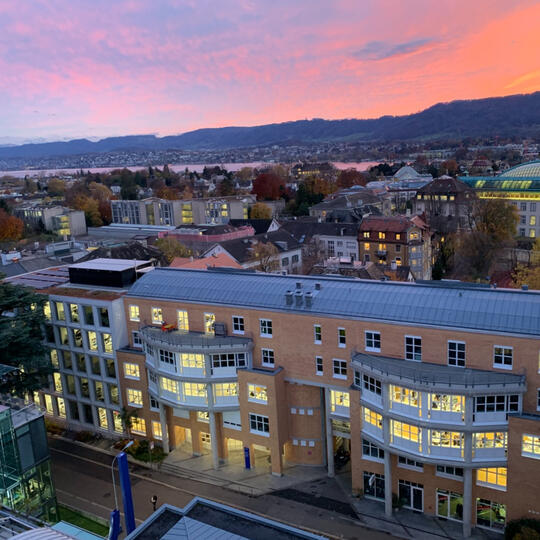